Leveraging Machine Learning for Business Growth
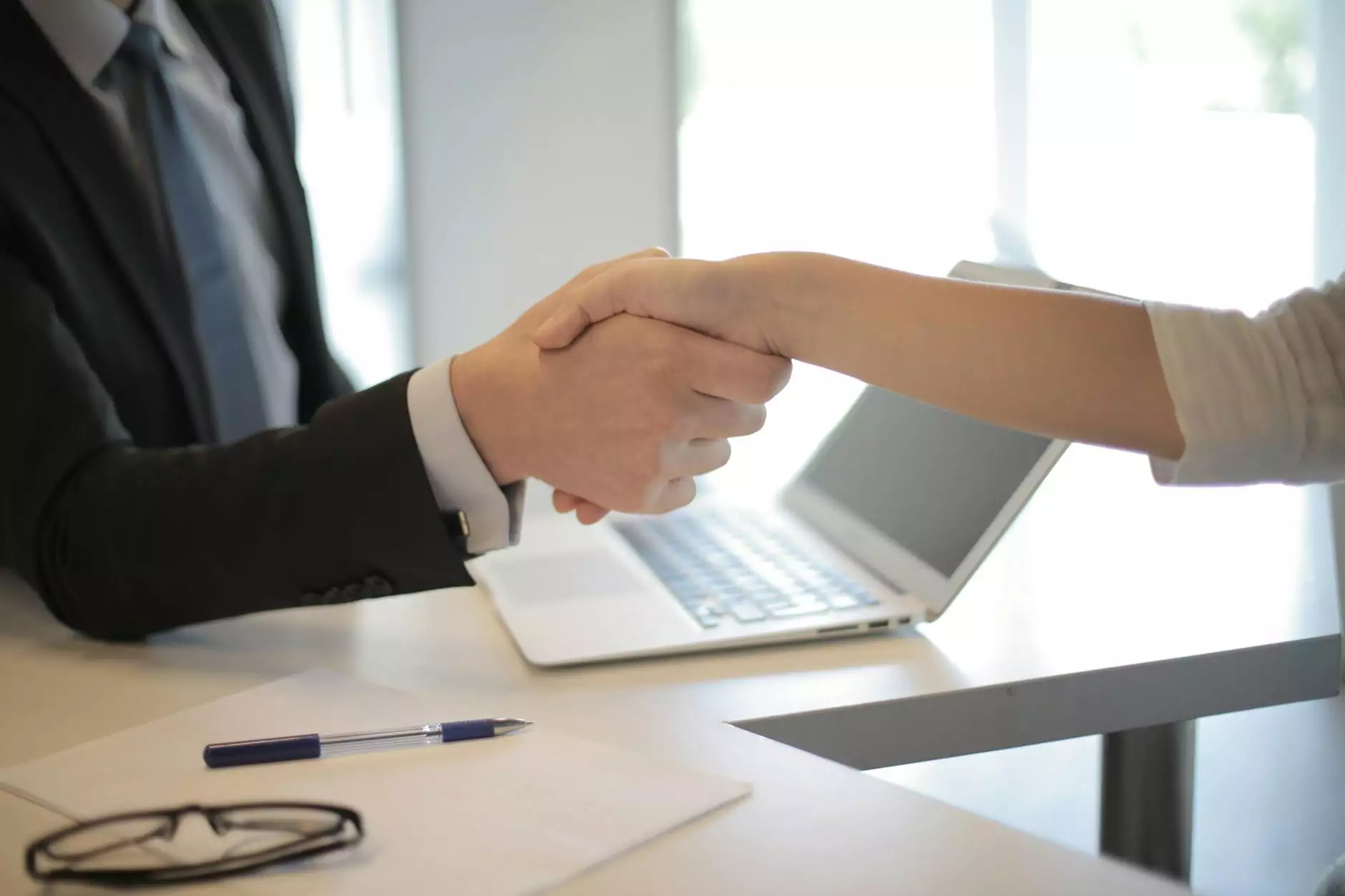
Machine learning has become a vital force in the dynamic landscape of modern business. In today’s fast-paced environment, organizations are increasingly turning to machine learning blog posts to tap into this revolutionary technology. By harnessing the power of data, machine learning facilitates smarter business decisions and enhances operational efficiency. In this comprehensive guide, we'll explore the multifaceted benefits of machine learning, how to implement it effectively, and the future trends that every business leader should be aware of.
Understanding Machine Learning
Machine learning refers to a subset of artificial intelligence (AI) that involves training algorithms to learn from and make predictions based on data. Unlike traditional programming, where rules are explicitly defined, machine learning systems improve their performance over time by analyzing patterns within data.
Types of Machine Learning
There are three primary types of machine learning:
- Supervised Learning: Involves training a model on a labeled dataset, enabling it to make predictions based on new, unseen data.
- Unsupervised Learning: Involves analyzing unlabeled data to uncover hidden patterns or groupings.
- Reinforcement Learning: Involves training a model to make decisions by rewarding it for achieving desired outcomes.
Benefits of Machine Learning for Businesses
Implementing machine learning can bring numerous advantages to businesses across various sectors. Here are some key benefits:
1. Enhanced Decision-Making
Data-driven decision-making is crucial for success in today's marketplace. By utilizing machine learning blog posts, companies can gain insights from large datasets to make informed decisions. Machine learning models can analyze historical data, recognize patterns, and predict future trends, allowing businesses to respond proactively to market changes.
2. Improved Customer Experience
Machine learning can significantly enhance customer interactions. By analyzing customer behavior and preferences, businesses can deliver personalized experiences. Recommendations engines, such as those used by e-commerce platforms, utilize machine learning to suggest products tailored to individual customer tastes, improving conversion rates and customer satisfaction.
3. Operational Efficiency
Automation is one of the most profound impacts of machine learning on business operations. Routine tasks, such as data entry, report generation, and even complex analytics can be automated, freeing up employees to focus on higher-level strategic initiatives. This not only speeds up processes but also reduces the potential for human error, leading to greater efficiency and accuracy.
4. Predictive Analytics
Predictive analytics is a powerful application of machine learning that allows businesses to anticipate trends and outcomes. By analyzing past performance data, companies can predict future sales, customer behavior, and other key metrics, enabling them to adapt strategies accordingly.
Implementing Machine Learning in Business
While the benefits are clear, successfully implementing machine learning requires careful planning and execution. Here are the steps to consider:
1. Identify Goals and Objectives
Begin by defining clear business objectives. What problems do you aim to solve with machine learning? Whether it’s improving product recommendations or streamlining supply chain logistics, having specific goals will guide the implementation process.
2. Data Collection and Preparation
Data is the foundation of machine learning. Gather data from various sources, ensuring that it is relevant, high-quality, and sufficient in volume. Data preparation, including cleaning and preprocessing, is critical to creating an effective machine learning model.
3. Choose the Right Tools and Technologies
Select appropriate machine learning frameworks and tools that align with your objectives. Popular options include:
- TensorFlow: An open-source framework developed by Google for building machine learning models.
- Scikit-learn: A Python library that provides simple and efficient tools for data mining and analysis.
- PyTorch: A deep learning framework that offers a flexible and easy-to-use platform for model building.
4. Model Training and Evaluation
Once the data is ready, you can begin training models using various algorithms. Assess the performance of these models through rigorous evaluation methods such as cross-validation, ensuring they meet the required accuracy and reliability before deployment.
5. Deployment and Monitoring
After testing, deploy the models into real-world applications. Continuous monitoring is crucial to ensure the models adapt and perform well as new data becomes available. Collect feedback and refine the models as necessary to maintain optimal results.
Challenges in Machine Learning Adoption
Despite its benefits, several challenges may hinder machine learning adoption:
1. Data Privacy and Security
Managing vast amounts of data raises concerns about privacy and security. Organizations must prioritize data governance and ensure compliance with regulations such as GDPR and CCPA to protect sensitive information.
2. Talent Gap
There is a significant demand for skilled data scientists and machine learning engineers. Companies may face challenges in hiring or training personnel with the right expertise to develop and maintain machine learning systems.
3. Integration with Existing Systems
Integrating new machine learning solutions with legacy systems can be complex. Companies must plan for seamless integration to fully leverage the capabilities of machine learning.
Future Trends in Machine Learning
The future of machine learning is incredibly promising. Here are some key trends to watch:
1. Enhanced Natural Language Processing (NLP)
NLP technologies are advancing rapidly, enabling machines to understand, interpret, and respond to human language. Applications such as chatbots and virtual assistants are becoming more sophisticated, improving customer interactions.
2. Automated Machine Learning (AutoML)
AutoML simplifies the machine learning process, making it accessible to non-experts. It automates various stages of model development, from data preprocessing to model selection, allowing businesses to implement machine learning more efficiently.
3. Ethical AI and Accountability
With the growing use of machine learning, the focus on ethical AI is intensifying. Businesses must ensure that their machine learning practices are fair, transparent, and accountable, addressing concerns about bias and discrimination.
Conclusion
In conclusion, machine learning is redefining the way businesses operate and compete. Through informed machine learning blog posts, organizations can gain valuable insights and strategies to leverage this powerful technology for growth. By understanding the fundamentals of machine learning, embracing its benefits, and navigating the challenges of implementation, businesses can position themselves at the forefront of innovation and success. As the landscape continues to evolve, staying abreast of emerging trends will be crucial for maintaining a competitive edge in an increasingly data-driven world.
As you embark on your machine learning journey, remember to leverage reliable resources, engage in continuous learning, and remain flexible to adapt to the rapidly changing technological landscape. The future is bright for those ready to embrace the power of machine learning.