Unlocking Business Potential Through Exceptional Data Labelling Services
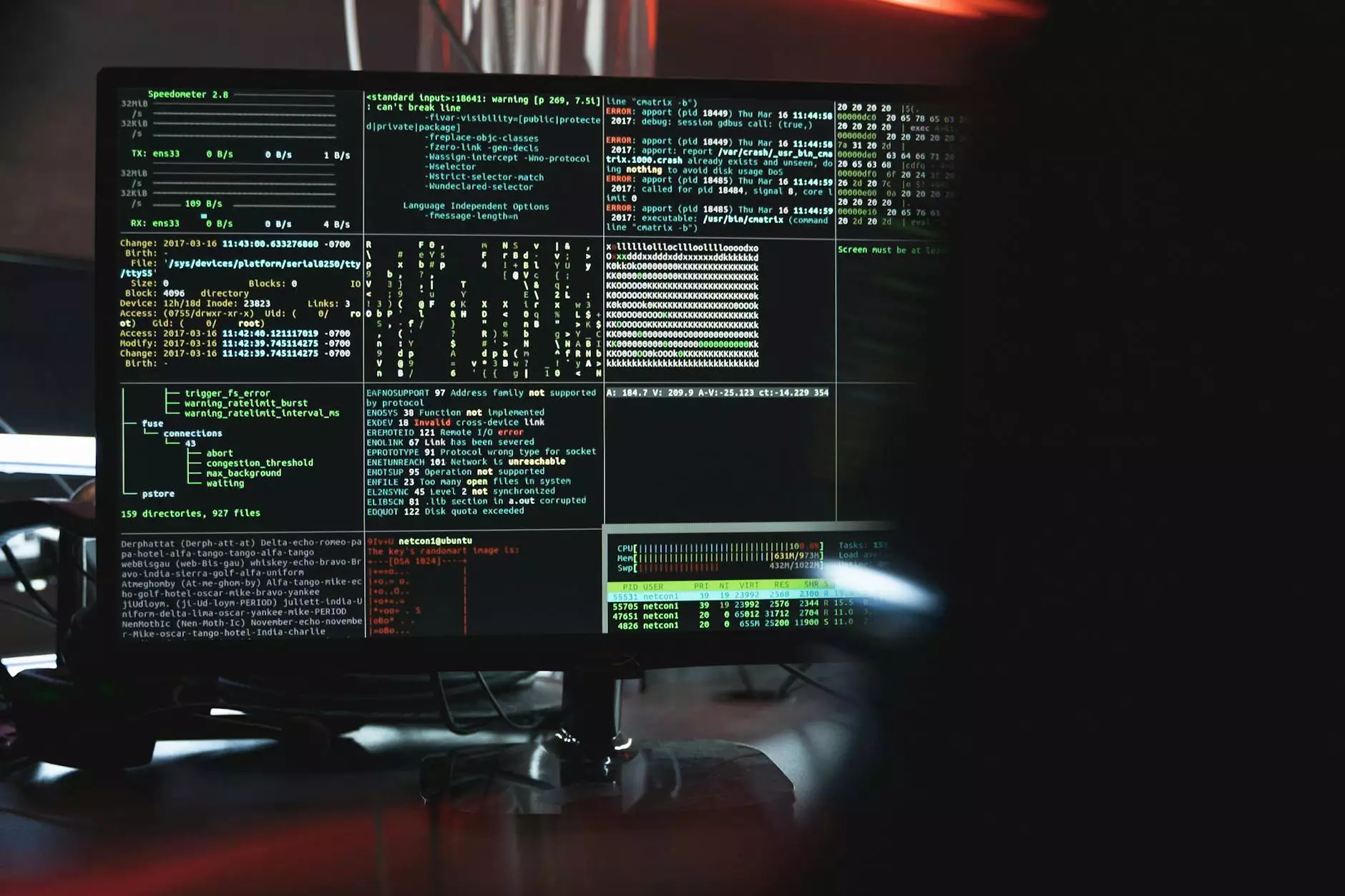
In today's data-driven world, businesses rely on high-quality datasets to train advanced artificial intelligence models and optimize operations. One of the critical components of this process is data labelling services, which involve the careful tagging and annotation of data to ensure accuracy and relevancy. As organizations increasingly turn to data annotation tools and platforms, understanding the benefits and intricacies of these services becomes paramount.
The Importance of Data Labelling Services
Data labelling is more than just a technical task; it is the foundation of machine learning and AI development. By providing structured and labeled data, organizations can:
- Improve Model Accuracy: High-quality labelled data boosts the performance of machine learning models, resulting in better predictions and insights.
- Enhance AI Training: Properly labelled datasets allow for effective training of AI systems, ensuring they learn the right patterns and features.
- Drive Business Efficiency: Automating processes based on clear and accurate data leads to streamlined operations and time savings.
- Support Informed Decision Making: With reliable datasets, businesses can make data-driven decisions, leading to improved strategies and outcomes.
How Data Labelling Services Work
The process of data labelling typically involves the following steps:
1. Data Collection
Data is collected from various sources, ranging from customer interactions to operational processes. This data can be in multiple forms, including text, images, audio, and video.
2. Annotation Guidelines
Clear annotation guidelines are established to ensure consistency and accuracy throughout the labelling process. Guidelines may include detailed instructions on what to tag, how to tag, and the significance of each label.
3. The Labelling Process
This is where the real magic happens. Trained data labelers, often using data annotation tools, meticulously annotate the datasets according to the established guidelines. This stage can be done manually, semi-automatically, or fully automatically, depending on the tools used.
4. Quality Assurance
Once the data has been labelled, a thorough quality assurance process ensures the labels' accuracy and consistency. This phase is crucial as it directly impacts the performance of AI models trained with the data.
5. Delivery of Annotated Data
After passing through quality checks, the labelled dataset is delivered to the client in the required format, ready for use in machine learning models or data analytics.
Types of Data Labelling Services
Data labelling services can be categorized based on the type of data being annotated. Here are some common types:
1. Image Annotation
This service involves tagging objects within images. Applications include:
- Object Detection: Identifying and locating objects within images.
- Image Segmentation: Dividing an image into segments to simplify analysis.
- Facial Recognition: Labelling facial features for identification purposes.
2. Text Annotation
In text annotation, data labelers tag segments of text with relevant labels. This can include:
- Sentiment Analysis: Identifying the sentiment expressed in a piece of text.
- Entity Recognition: Tagging entities like names, dates, and locations.
- Intent Detection: Classifying user intent based on text input.
3. Audio and Video Annotation
Audio and video data labelling services focus on transcribing and labeling audio input and highlighting significant events in videos. Some applications include:
- Speech Recognition: Converting spoken language into text.
- Activity Recognition: Labeling actions or events in video footage.
- Emotion Detection: Analyzing emotional tone in audio responses.
Choosing the Right Data Annotation Platform
When it comes to selecting a data annotation platform, several factors should be considered to ensure it meets your business needs:
1. Accuracy and Quality
The primary goal of any data labelling service is to provide accurate and high-quality annotations. Look for platforms that prioritize quality assurance processes and have positive reviews from clients.
2. Scalability
Your data labelling needs may vary over time. Choose a platform that can scale with your business, accommodating small projects and larger datasets as necessary.
3. Variety of Annotation Tools
Different projects may require different annotation approaches. A good data annotation platform should offer a variety of tools for image, text, audio, and video annotation.
4. Security and Compliance
Data privacy is vital, especially in industries dealing with sensitive information. Ensure the platform complies with industry standards and provides robust data security measures.
5. Customization
Every business has unique requirements. Look for platforms that allow customization of annotation guidelines and workflows to suit specific project needs.
Integrating Data Labelling Services into Your Business Workflow
Implementing data labelling services can significantly enhance your AI initiatives. Here’s how to effectively integrate these services into your workflow:
1. Identify Data Needs
Assess your current data assets and identify gaps where labelling can add the most value. This assessment should align with your business objectives and data strategy.
2. Collaborate with Experts
Leverage the expertise of data annotators who understand your industry. Collaboration ensures that the labelling process meets specific standards and requirements.
3. Utilize the Right Tools
Adopt advanced data annotation tools that streamline the labelling process and improve productivity. Invest in training for your team to effectively use these tools.
4. Continuously Monitor and Evaluate
Regularly review the quality of labelled data and the performance of AI models. Continuous evaluation helps identify areas for improvement and adjustment.
The Future of Data Labelling Services
As businesses increasingly embrace AI and machine learning technologies, the demand for high-quality data labelling services will only continue to grow. Trends influencing the future of data annotation include:
- Automation: The integration of automation in data labelling processes will enhance efficiency, although human oversight will remain essential for quality assurance.
- AI-Powered Annotation: Machine learning tools are being developed to assist in the annotation process, reducing the time and effort required.
- Standardization: As the industry matures, there will be a push towards standardizing data labelling practices and creating universal guidelines for various data types.
Conclusion
In conclusion, data labelling services play a crucial role in empowering businesses to harness the full potential of their data. By investing in high-quality data annotation tools and platforms, organizations can enhance their AI models, drive better decision-making, and improve overall operational efficiency. Choose a reputable provider like Key Labs to ensure you have the best resources at your disposal for your data labelling needs. Embrace the power of data, and watch your business transform into a more efficient, data-driven enterprise.