Understanding Machine Learning Data Labeling: A Key Component for Business Success
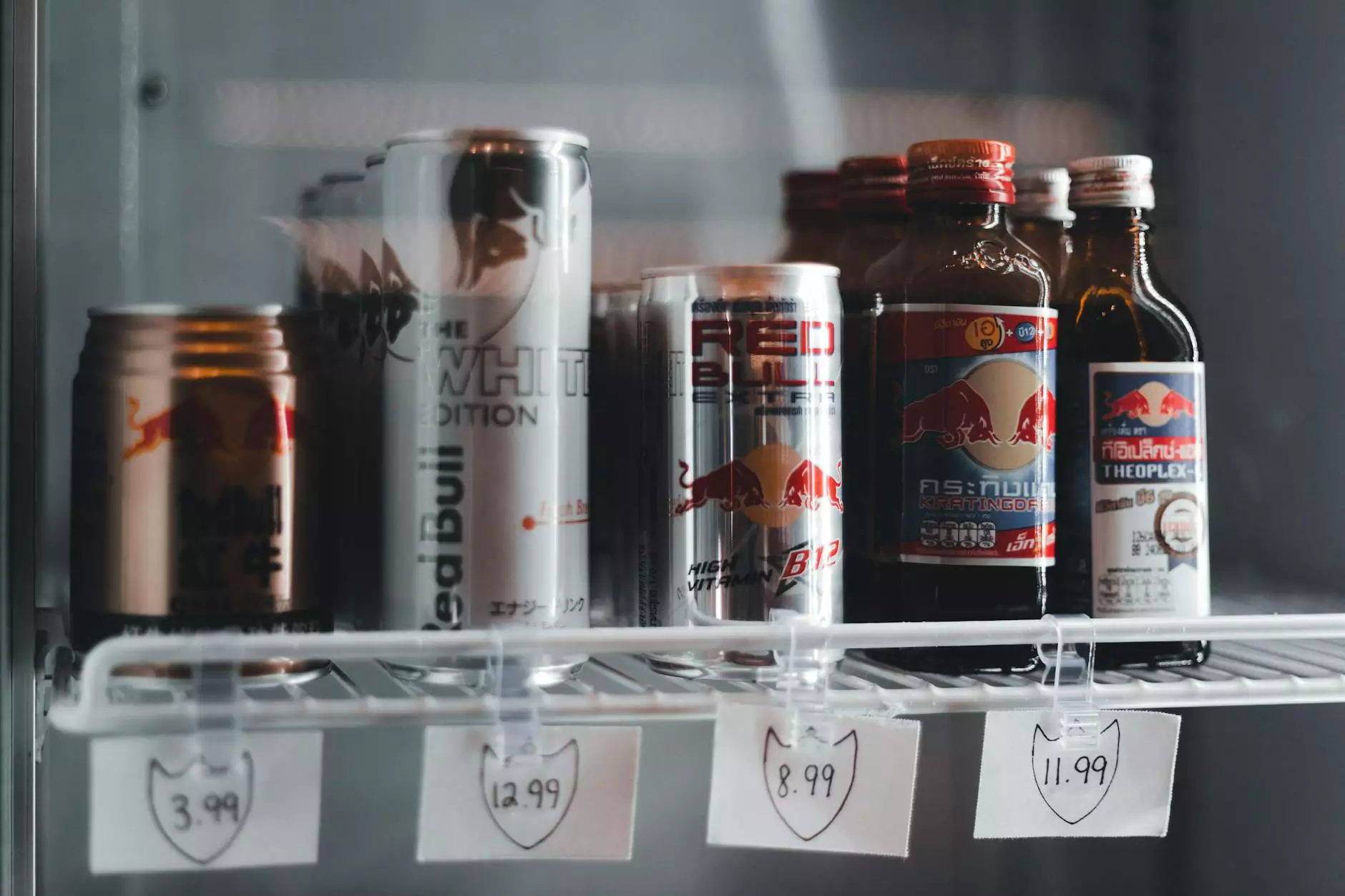
Machine learning data labeling has emerged as a crucial element in the development of artificial intelligence (AI) systems. It serves as the backbone for training algorithms, enabling them to learn from data and make informed decisions. In industries like Home Services and Keys & Locksmiths, leveraging machine learning can dramatically elevate the quality of service and operational efficiency. In this article, we will explore the importance of data labeling, its process, and its potential benefits for businesses.
The Fundamentals of Machine Learning Data Labeling
Data labeling involves the process of annotating data sets to provide them with meaningful labels. These labels help machine learning models identify patterns and make accurate predictions. For instance, in the field of locksmithing, data labeled with specific lock types, vulnerabilities, or service requests can lead to more refined algorithms that enhance security solutions.
Why is Data Labeling Important?
The significance of data labeling lies in its direct correlation with the performance of machine learning models. Here are some key reasons why it’s indispensable:
- Improved Accuracy: Labeled data allows algorithms to understand inputs better, leading to improved prediction accuracy.
- Quality Insights: Accurate data labeling facilitates insightful analysis, helping businesses make strategic decisions.
- Faster Deployment: Well-labeled data speeds up the training process, allowing businesses to deploy machine learning solutions more quickly.
- Enhanced Customer Experience: In home services, understanding customer needs through data can tailor services effectively, improving customer satisfaction.
The Process of Data Labeling
The process of machine learning data labeling involves several stages that ensure the data is accurately annotated for training models:
- Data Collection: Gather relevant data from diverse sources. This could include images, text, or other data types relevant to your business.
- Data Preprocessing: Clean and prepare your data by removing duplicates or irrelevant information to maintain high quality.
- Annotation: Use skilled annotators or automated tools to assign labels to the data. This could range from simple categorizations to complex tagging.
- Quality Assurance: Implement a quality control process to review the labeled data, ensuring accuracy and consistency.
- Model Training: Utilize the labeled data to train your machine learning models, allowing them to learn and improve from real-world scenarios.
Types of Data Labeling
Data labeling can take various forms depending on the type of data being utilized:
- Image Labeling: Useful in locksmithing for identifying lock types or distinguishing between various home services visually.
- Text Classification: Effective for categorizing customer inquiries or service requests in the home services sector.
- Semantic Segmentation: Important for understanding the context of images, which can assist in training algorithms for identifying specific features in locksmithing.
Applications of Machine Learning in Home Services and Locksmithing
The application of machine learning in Home Services and Keys & Locksmiths is transforming the way businesses operate. Here are several ways how:
Predictive Maintenance
Machine learning models trained with labeled data can predict when equipment is likely to fail. This allows companies to perform maintenance proactively, saving costs and improving service reliability.
Customer Insights and Service Personalization
By analyzing labeled customer data, businesses can understand patterns in service usage. This insight aids in crafting personalized marketing strategies, enhancing customer satisfaction, and ultimately driving loyalty.
Smart Security Systems
For locksmiths, integrating machine learning can lead to innovations in security systems. Labeled data about different security threats can help create smarter locks and alarm systems that adapt to emerging crime trends.
The Future of Machine Learning Data Labeling
As AI technologies continue to advance, the future of machine learning data labeling looks promising. Companies can expect:
- Automation: The rise of sophisticated automated data labeling tools will ease the workload, allowing employees to focus on more strategic tasks.
- Enhanced Data Quality: With advances in machine learning, data quality assurance processes will automatically flag and correct labeling errors, increasing reliability.
- Integration of Crowdsourcing: Crowdsourcing will play a vital role in efficiently gathering and labeling massive data sets, enhancing cost-effectiveness.
Conclusion
In conclusion, the role of machine learning data labeling in the modern business landscape cannot be overstated. Particularly in the domains of Home Services and Keys & Locksmiths, embracing this technology unlocks a plethora of opportunities for growth, efficiency, and enhanced customer satisfaction. As businesses invest in quality data labeling processes, they pave the way for sophisticated machine learning applications that will shape the future of their operations. Harnessing this power ensures that companies can thrive in an increasingly data-driven world, staying ahead of competitors and meeting the evolving needs of their customers.
KeyMakr, a leader in the locksmithing sector, stands to benefit immensely from integrating machine learning data labeling into their systems. By enhancing their service quality and operational efficiency, they can truly revolutionize the locksmithing experience.